Research Projects on Computer Vision
- Reverse Pass-Through VR with Full Head Avatars
- High Resolution Solar Image Generation using Generative Adversarial Networks
- HierGAN: GAN-Based Hierarchical Model for Combined RGB and Depth Inpainting
- Attentive Partial Convolution for RGBD Inpainting
Reverse Pass-Through VR with Full Head Avatars
Virtual Reality (VR) headsets are becoming increasingly integral to today’s digital ecosystem, yet they introduce a critical challenge by obscuring users' eyes and portions of their faces, which diminishes visual connections and may contribute to social isolation. To address this, we introduce RevAvatar, a device-agnostic framework designed to mitigate VR-induced isolation and enhance user interaction within both virtual and physical environments. RevAvatar enables real-time reverse pass-through by projecting users' eyes and facial expressions onto VR headsets. The end-to-end system leverages deep learning and computer vision to reconstruct 2D facial images from partially observed eye and lower-face regions captured by VR headsets, while also generating accurate 3D head avatars using a one-shot approach for VR meetings and other immersive experiences. Its real-time capabilities ensure rapid inference and seamless deployment across mainstream VR devices. To further support research in this area, we introduce VR-Face, a novel dataset comprising 70,000 samples that simulate complex VR conditions, including diverse occlusions, lighting variations, and differing levels of visibility.
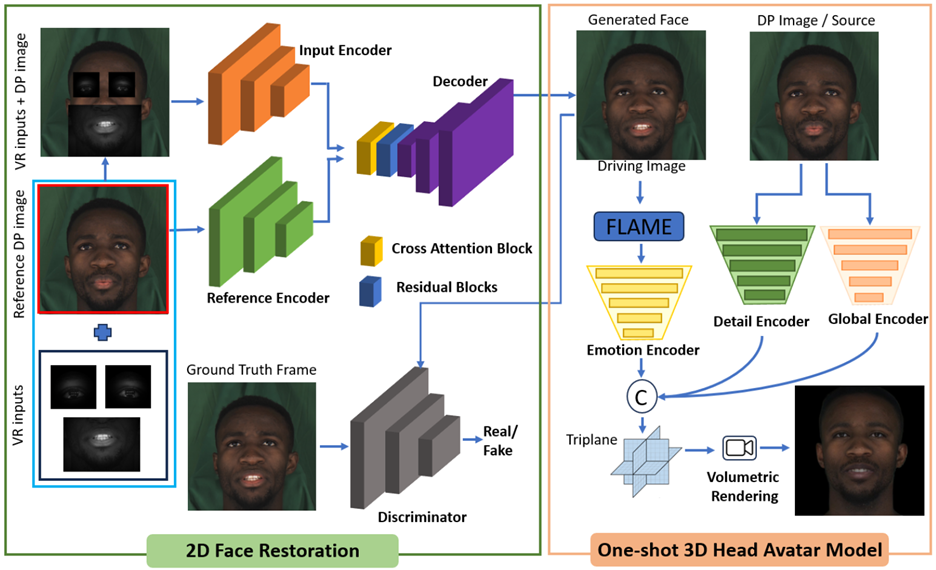
High Resolution Solar Image Generation using Generative Adversarial Networks
We applied Deep Learning algorithm known as Generative Adversarial Networks (GANs) to perform solar image-to-image translation. That is, from Solar Dynamics Observatory (SDO)/Helioseismic and Magnetic Imager(HMI) line of sight magnetogram images to SDO/Atmospheric Imaging Assembly(AIA) 0304-Å images. Ultraviolet (UV)/Extreme Ultraviolet(EUV) observations like the SDO/AIA0304-Å images were only made available to scientists in the late 1990s even though magnetic field observations like the SDO/HMI have been available since the 1970s. Therefore by leveraging Deep Learning algorithms like GANs we can give scientists access to complete datasets for analysis. For generating high resolution solar images we use the Pix2PixHD and Pix2Pix algorithms. The Pix2PixHD algorithm was specifically designed for high resolution image generation tasks, and the Pix2Pix algorithm is by far the most widely used image to image translation algorithm. For training and testing we used the data for the year 2012, 2013 and 2014. The results show that our deep learning models are capable of generating high resolution(1024 x 1024 pixels) AIA0304 images from HMI magnetograms. Specifically, the pixel-to-pixel Pearson Correlation Coefficient of the images generated by Pix2PixHD and original images is as high as 0.99. The number is 0.962 if Pix2Pix is used to generate images. The results we get for our Pix2PixHD model is better than the results obtained by previous works done by others to generate AIA0304 images. Thus, we can use these models to generate AIA0304 images when the AIA0304 data is not available which can be used for understanding space weather and giving researchers the capability to predict solar events such as Solar Flares and Coronal Mass Ejections. As far as we know, our work is the first attempt to leverage Pix2PixHD algorithm for SDO/HMI to SDO/AIA0304 image-to-image translation.
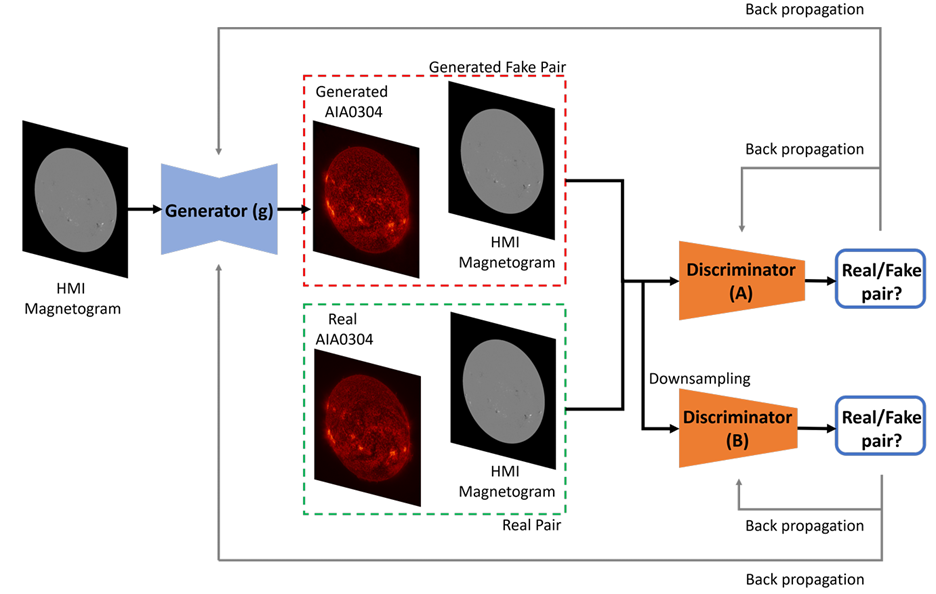
HierGAN: GAN-Based Hierarchical Model for Combined RGB and Depth Inpainting
Inpainting involves filling in missing pixels or areas in an image, a crucial technique employed in Mixed Reality environments for various applications, particularly in Diminished Reality (DR) where content is removed from a user’s visual environment. Existing methods rely on digital replacement techniques, which necessitate multiple cameras and incur high costs. AR devices and smartphones use ToF depth sensors to capture scene depth maps aligned with RGB images. Despite their speed and affordability, ToF cameras create imperfect depth maps with missing pixels. To address these challenges, we propose Hierarchical Inpainting GAN (HierGAN), a novel approach comprising three GANs in a hierarchical fashion for RGBD inpainting. EdgeGAN and LabelGAN inpaint masked edge and segmentation label images, respectively, while CombinedRGBD-GAN combines their latent representation outputs and performs RGB and depth inpainting. Edge images and particularly segmentation label images as auxiliary inputs significantly enhance inpainting performance by providing complementary context and hierarchical optimization. We believe we are the first to incorporate label images into the inpainting process. Unlike previous approaches requiring multiple sequential models and separate outputs, our work operates in an end-to-end manner, training all three models simultaneously and hierarchically. Specifically, EdgeGAN and LabelGAN are first optimized separately and further optimized within CombinedRGBD-GAN to enhance inpainting quality. Experiments demonstrate that HierGAN works seamlessly and achieves overall superior performance compared to existing approaches.
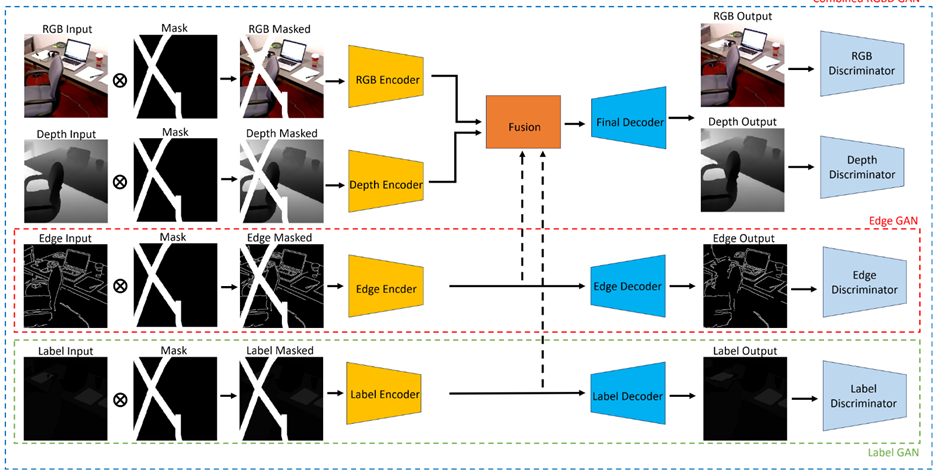
Attentive Partial Convolution for RGBD Inpainting
The process of Inpainting, which involves reconstructing missing pixels within images, plays a pivotal role in refining image processing and augmenting reality (AR) encounters. This study tackles three prominent hurdles in AR technology: diminished reality (DR), which entails removing undesired elements from the user's view; the latency issue in AR head-mounted displays leading to pixel gaps; and the flaws in depth maps generated by Time-of-Flight (ToF) sensors in AR devices. These obstacles compromise the authenticity and engagement of AR experiences by affecting both the texture and geometric accuracy of digital content. We introduce an innovative Partial Convolution-based framework tailored for RGBD (Red, Green, Blue, Depth) image inpainting, proficient in simultaneously reinstating missing pixels in both the color (RGB) and depth dimensions of an image. Unlike traditional methods that primarily concentrate on RGB inpainting, our approach integrates depth data, crucial for lifelike AR applications, by restoring both the spatial structure and visual details. This dual restoration ability is paramount for crafting immersive AR experiences, ensuring seamless amalgamation of virtual and real-world elements. Our contributions encompass the refinement of an advanced Partial Convolution model, incorporating attentive normalization and an updated loss function, which surpasses existing models in accuracy and realism in inpainting endeavors.
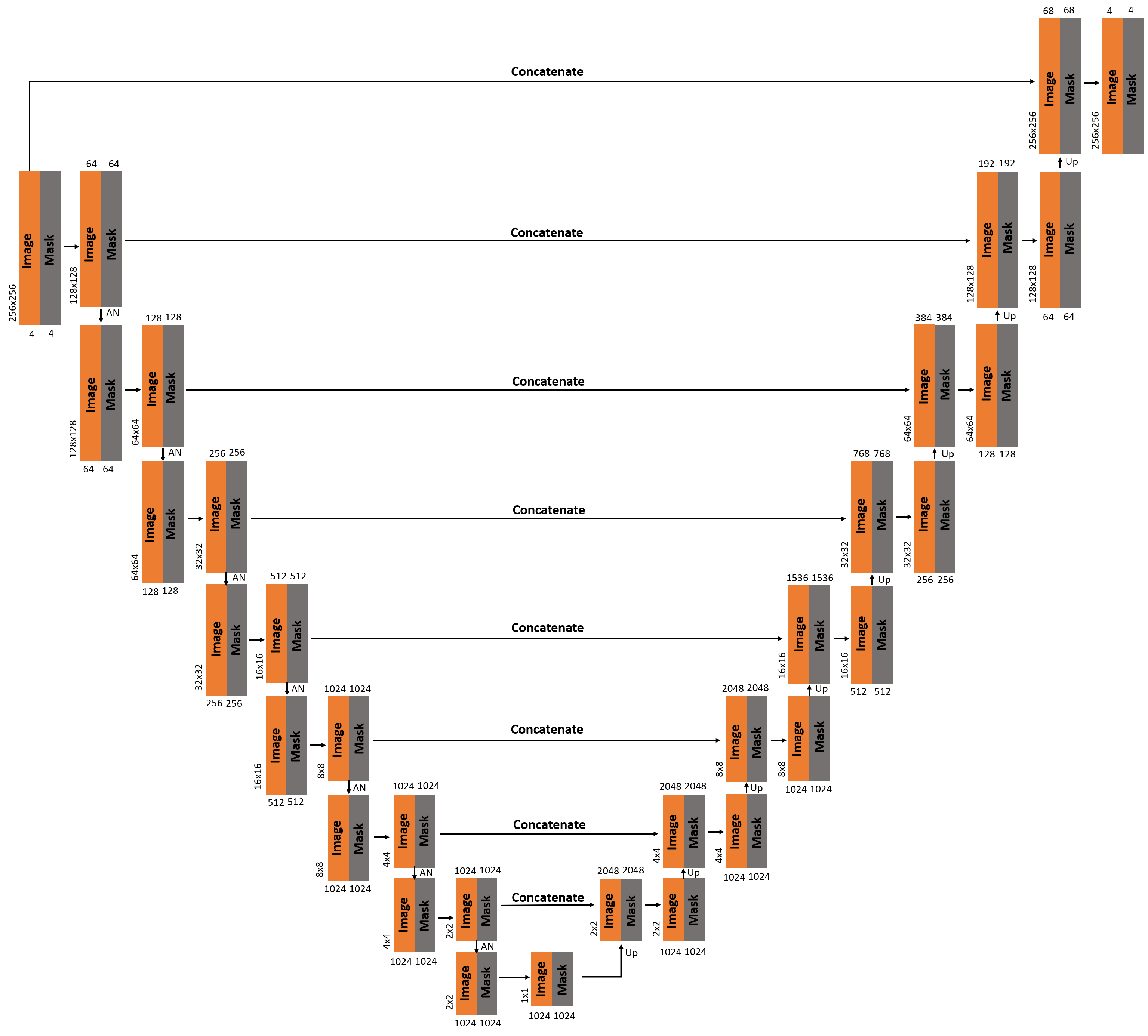