According to reports of Interactive Advertising Bureau (IAB), online display advertising has emerged as the largest medium after surpassing TV broadcast advertising since 2013 (IAB 2014). The revenue of digital advertising in 2015 reached to 59.6 billion dollars in United States, 20.4% higher than in 2014 (IAB 2016). As a massive industry, display advertising delivers advertisers' marketing messages to attract customers through graphic banners on webpages. By investing in online display advertising, advertisers can efficiently promote their brand awareness and encourage an audience of interest to take some actions, e.g. purchase. On the other side of the table, today display advertising is the most critical revenue source for publishers. Personalized ads can also help users to find relevant products. While studies have been performed from the advertisers' side about how to provide tailored ads to users, there are little studies on computational advertising from the publishers' side and on the relationships between publishers and users.
The goal of our research is to understand the economics and behaviors in online display advertising by developing computational models and machine learning techniques to analyze big historic log data. This research will contribute to a healthy ecosystem of online advertising, in order to sustain the free Web.
Several issues that we are currently studying are listed below.
Ad-Blocking: The fast increase of ad blocker usage significantly impacts publishers' and advertisers'. In response, a growing number of online publishers initialize their counter-ad blocking strategies, e.g. ad-blocking wall, which prevents ad blocker users to read the content unless they whitelist the website. While these counteraction may suppress the use of ad blocker on the website, it may also result in the loss of user population and user traffic. We are studying the factors related ad blocker usage, such as user profile, device features and behavior patterns, by analyzing big data from real-world applications (pdf).
Viewability Prediction. In display advertising, advertisers use online display ads to raise brand awareness and to promote the visibility of the companies and their products. However, a recent study shows that more than half of all impressions are actually not viewed by users because they do not scroll down a page enough to view the ads. Thus, a new pricing model is emerging: pricing ads by the number of impressions that can be viewed by a user, instead of just being served. For instance, an industry standard of viewable impressions, as developed by the Media Rating Council (MRC), calls for display ads to be viewable if 50% of their pixels are in-view for a minimum of one consecutive second. With this new pricing standard, it is important to predict whether or not an ad in a particular page location will be viewed in order to determine which ads to serve to satisfy advertisers' contractual requirements and to maximize publishers' revenue. Predicting whether a particular page location will be viewed by a user can also help us to understand user interests for better serving content.
In this research, we propose machine learning models to predict ad viewability in real-time from two perspectives: 1) scrolling behavior prediction, i.e. what is the page depth that a specific user may scroll to, on a specific page (link,link), and 2) page depth-level dwell time prediction, i.e. how long a user will view the content at a specific page depth (link).
Recommender Systems. Publishers strive for extending the user population and increasing traffic by providing high-quality content and recommending the most relevant content to users. On the other hand, publishers would like to maximize the revenue received from each individual page view through recommending profitable pages. The challenge is how to design a recommender system to recommend webpage articles which are interesting to specific users, and at the same time, can bring large revenue to the publisher to sustain the free Web. We are studying how to develop a long-term revenue-optimized recommender system (RORS) that recommends webpages of interest to the user to sustain and increase traffic at the long term, and at the same time, with optimized ad revenue on current page view (link).
Achir Kalra, Chong Wang, Cristian Borcea, Yi Chen. Reserve Price Optimization in First-Price Auctions via Multi-Task Learning In Proceedings of the 2023 IEEE International Conference on Data Mining (ICDM), 2023.
Shuai Zhao, Michael K. Chen, Cristian Borcea, Yi Chen. Personalized Dynamic Counter Ad-Blocking Using Deep Learning IEEE Transactions on Knowledge and Data Engineering (TKDE), 2022.
Shuai Zhao, Achir Kalra, Cristian Borcea, Yi Chen. To be Tough or Soft: Measuring the Impact of Counter-Ad-blocking Strategies on User Engagement In Proceedings of The Web Conference 2020, 2020.
Shuai Zhao, Achir Kalra, Chong Wang, Cristian Borcea, Yi Chen. Ad Blocking Whitelist Prediction for Online Publishers In Proceedings of the 2019 IEEE International Conference on Big Data (Big Data), 2019.
Achir Kalra, Chong Wang, Cristian Borcea, Yi Chen. Reserve Price Failure Rate Prediction with Header Bidding in Display Advertising In Proceedings of the 25th ACM SIGKDD International Conference on Knowledge Discovery & Data Mining (KDD), 2019.
Chong Wang, Achir Kalra, Li Zhou, Cristian Borcea, Yi Chen. Webpage Depth Viewability Prediction Using Deep Sequential Neural Networks IEEE Transactions on Knowledge and Data Engineering (TKDE), 2018.
Chong Wang, Shuai Zhao, Achir Kalra, Cristian Borcea, Yi Chen. Predictive Models and Analysis for Webpage Depth-Level Dwell Time Journal of the Association for Information Science and Technology (JASIST), 2018.
Chong Wang, Achir Kalra, Li Zhou, Cristian Borcea, Yi Chen. Probabilistic Models for Ad Viewability Prediction on the Web IEEE Transactions on Knowledge and Data Engineering (TKDE), 2017.
Shuai Zhao, Chong Wang, Achir Kalra, Leon Vaks, Cristian Borcea, Yi Chen. Ad Blocking and Counter-Ad Blocking: Analysis of Online Ad Blocker Usage In Proceedings of the 2017 Americas Conference on Information Systems (AMCIS), 2017.
Chong Wang, Achir Kalra, Cristian Borcea, Yi Chen. Webpage Depth-level Dwell Time Prediction In Proceedings of the 25th ACM International on Conference on Information and Knowledge Management (CIKM), 2016.
Chong Wang, Achir Kalra, Cristian Borcea, Yi Chen. Revenue-Optimized Webpage Recommendation. In Proceedings of the 2015 IEEE International Conference on Data Mining Workshop (ICDMW), 2015.
Chong Wang, Achir Kalra, Cristian Borcea, Yi Chen. Viewability Prediction for Online Display Ads. In Proceedings of the 24th ACM International on Conference on Information and Knowledge Management (CIKM), 2015.
Faculty:
Yi Chen < yi.chen@njit.edu >
Cristian Borcea < borcea@njit.edu >
Alumni:
Shuai Zhao (Business Data Science)
Chong Wang (Information Systems)
Achir Kalra (Computer Science, Forbes)
Participants:
Leon Vaks
Collaborator:
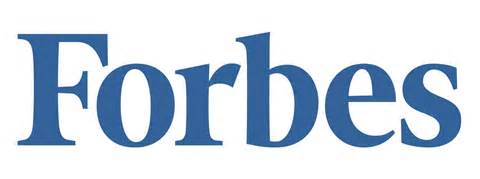
This project is supported by a NJIT seed grant.