The
purpose of this project is developing and delivering clean
renewable energy to homes and business in collaboration with
Cattron, Stanley Vidmar, Bolaball and
I.D. Systems, INC. Bio-Green
Alternative Energy Solutions is
intended to replace fuel sources without the undesired
consequences of the fuels.
Our company’s mission is
bringing innovative ways to cover the need to overcome the
shortage in energy by developing secure energy solutions that
are sustainable and are technologically and financially viable.
Our
experience in industry gives us a deep insight into the
technical, policy, economic, and regulatory challenges being
faced by our energy clients. We help clients view energy issues
in a broader context, and to manage energy strategically to
ensure that their clean energy and energy reduction solutions
grow with the organization.
For many
years mankind has been looking for an alternative source of
electrical power. Presently, high oil prices make the
investment for this new alternative viable. This is needed to
solve the continuing pollution resulting from burning fossil
fuels and from nuclear waste.
In order
to monitor and control the wind percentage in turbines and
reduce its power consumption Bio-Green Alternative Energy implements controls to achieve
maximum performance in manufacture and installation of superior
quality wind turbines by using control charts for variables and
attributes. These charts are one of the most useful tools when
studying variation. They offer a method that determines whether
a process is stable or unstable which enables the user to modify
when unstable or control the process if already stable. This can
be accomplished by sampling of a particular process, analyzing
the results; assign a cause and taking any needed actions.
The
turbines are designed for maximum durability in sustained high
wind environments with multi-voltage controller is included and
excess power is diverted through a resistor network that can
also be used for area heating.
(SPC) is
an effective method of monitoring a process through the use of
control charts. Control charts enable the use of objective
criteria for distinguishing background variation from events of
significance based on statistical techniques. Much of its power
lies in the ability to monitor both process center and its
variation about that center. By collecting data from samples at
various points within the process, variations in the process
that may affect the quality of the end product or service can be
detected and corrected, thus reducing waste as well as the
likelihood that problems will be passed on to the customer. With
its emphasis on early detection and prevention of problems, SPC
has a distinct advantage over quality methods, such as
inspection, that apply resources on detecting and correcting
problems at the end product or service one.
In addition to reducing waste, SPC can lead to a reduction in
the time required to produce the product or service from end to
end. This is partially due to a diminished likelihood that the
final product will have to be reworked, but it may also result
from using SPC data to identify bottlenecks, wait times, and
other sources of delays within the process. Process cycle time
reductions coupled with improvements in yield have made SPC a
valuable tool from both a cost reduction and a customer
satisfaction standpoint two.
Collaborating Companies
1.
Stanley Vidmar Tough Storage Solutions:
2.
Bolaball INC:
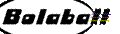
3.
Cattron
Group International:
4. ID Systems INC:
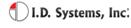
Control
charts for variable were developed by Dr. Walter A. Shewhart
while working for Bell Telephone labs in 1920’s. These Variable
Control Charts is a statistical tool to determine if a process
is in control that deal with items that can be measured, such as
height, weight, speed, and volume. The reasons for using control
charts, is because it improves productivity, make defect
visible, determines what process adjustments need to be made,
and determines if process is in or out control. To calculate the
major lines in a control chart, we can:
 |
Take the
average value by taking the average of the sample data,
|
 |
UCL: Multiply
the standard deviation by three, and then add the value to the
average value |
 |
LCL: Multiply
the standard deviation by three and then subtract that value
from the average value. |
UCL,
LCL (Upper and Lower Control Limit)
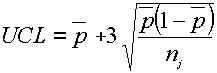
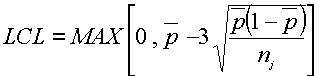
X-bar Chart:
1. Lower
Control Limit:
Mean –
3*sigma
n(1/2)
2. Center
Line:
Process mean
3. Upper
Control Limit:
Mean +
3*sigma
n(1/2)
R chart:
Lower Control Limit:
R-Bar – 3*d3*sigma
Center Line:
R-Bar
Upper Control Limit:
R-Bar + 3*d3*sigma
Here is how we
can interpret the data. First, the study always starts with the
R-chart (bottom right hand corner of the excel document). If the
R-chart shows a process in control, then the X bar chart can be
analyzed, but not before. There are typically two conditions to
check the status.
Condition 1
-
There are out of control upper and lower limits. If they are not
exceeded, then the process is in control (so far), we then check
the next condition.
Condition 2
- No
points are out of limits. Two sub-conditions have to be checked:
1. If there are
seven consecutive points above or below the central line.
2. If there are
seven consecutive points increasing or decreasing it detects
non-random patterns and shows that the process is out of
control.
If none of
these rules applies to the chart, then the process is still in
statistical control.
As part of our
Eco-friendly approached, our recycle process will use
microcontrollers and sensors in order to select waste plastic
material less than five pounds and more than five pounds. With
this process can select the amount of head that can be added in
the melting process.

R-Chart1
As we can see,
in the R chart, there are more than seven consecutive points
above the central line; we can call this process out-of-
control. Since the first condition was met, we can observe the
X-bar Chart
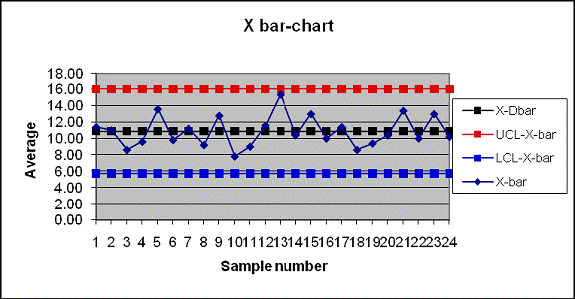
X-Bar -Chart
As we observed,
the x-Bar is in control.
After review
the R-Chart, we can see that from 2 to 9 sample number, there is
more than five consecutive points above the central line, it
means that there is a problem to detect raw material over or
less five pounds, we concluded that the process is out of
control. After we analyzed back, we investigated the process
during this period and we were able to determine the most
important reasons why the process was out-of-control;
1- The scale
that was measuring the weight of the raw material was out scale,
it means that it was off by 3 pounds. It was producing that the
scale were taking more than 2 pound raw material as a five
pounds.
2-Tthe scale
was not place on the right position. It was off by a ¾ inches.
3-Some of
sensors were cover with dust so they didn’t detect the raw
material when it was coming on the coverable belt. The sensors
could not tell the scale that a piece of material was coming and
it could not provide a valid weight.
4- Some of the
micro controllers were misaligned. The controllers did not
prevent the process to continue sorting wrong weight plastic
material.
After correct
all the problems, this is a new R-chart that we get a new
“in-control process”
R-Chart 2
Attributes are
a very important quality check as it verifies if a particular
aspect of a product is defective. If the attribute being
measured is over the certain limit (UCL) for a majority of the
samples, then the process is out of control. The attributes need
to remain between the UCL and LCL to be acceptable or in
control.
The attribute
being measured is defective buttons in the ADA (Advanced Digital
Assistant).
1. 100 Samples
are selected at random from a batch. 2. Test is performed for 20
batches. 3. The number of units with defects is recorded.
Analysis
Summary Attributes Control Chart – We tested twelve samples of
100 units. Judging from the p-chart data and u-chart data,
nothing is out of control. The average failure is 3 out of 100,
which is a 97% pass rating It indicates that the process was
under control.
However, If any
point was out of control, we would have to investigate why this
is so. Once we can assign a reason to that point, we will
identify the source of the variability and correct the situation
and repeat the sampling process to bring the process in control.
 |
Total Quality Management (e-book), CIMware USA, Inc. &
CIMware Ltd. UK |
 |
Research Supplements provided by Prof. Paul G. Ranky
|
 |
Unique TQM e-Learning Pack, prepared by Prof. Paul G. Ranky
|
 |
CIMware USA website,
http://cimwareukandusa.com |
Control Chart Templates, by Prof. Ranky
Microsoft Excel 2003
Actual spreadsheet files for control charts for variables:
These are the template file used with my own data:
|